Generative AI คืออะไร และมาเกี่ยวข้องกับ Medical education ได้อย่างไร ลองมาค้นหาคำตอบกัน
Top 10 AI Tools (February 2024) 1
Name | Category | Description | |
---|---|---|---|
1 | ChatGPT(incl. Dall-E) | GPT | |
2 | Bing AI | GPT | Integrates ChatGPT into Bing search |
3 | Grammarly AI | Writing & Editing | Checks writing in real-time and coaches for improvements |
4 | Character.AI | Social & Characters | Lets you chat with over 18 million AI Characters |
5 | Bard | GPT | Google’s version of ChatGPT |
6 | Brainly (Homework Help) | Education | Helps students with their homework based on a photo of the exercise. |
7 | CourseHero (Tutoring) | Education | Lets students upload or select a textbook they need help with. |
8 | Replit | Programming/Coding | Helps programmers create and deploy web applications |
9 | TurnItIn (AI Detection) | Education | Helps teachers detect AI content in students’s homework |
10 | DeepAI | Image Generator | Creates images from prompts, alongside other AI tools |
จากตารางจะเห็นได้ว่า ChatGPT ของ OpenAI ก็ยังคงเป็นตัวชูโรงในลำดับต้น ตามมาด้วย AI เฉพาะทางสำหรับงานต่างๆ แต่ถ้าสังเกตจะเห็นได้ว่า GPT หรือ Generative pre-trained transformer จะได้รับความนิยมอย่างมาก
Generative Pre-trained transformer คือประเภทหนึ่งของ Large language model (LLM) มีพื้นฐานจาก transformer architecture และถูก pre-trained โดยข้อมูลขนาดใหญ่ ทำให้สามารถสร้างข้อมูลคล้ายมนุษย์ได้ 2
นอกจากความนิยมที่สูงจากผลการสำรวจ ChatGPT ยังสามารถแซง Social Media Platform เช่น Netflix และ Pinterest ในด้านการเข้าถึงเว็บไซต์ (traffic) ดังภาพด้านล่าง
แนวโน้มผู้คนใน Generation ใหม่ๆ ก็ไปในแนวทางเดียวกัน คือ สามารถเข้าถึง AI ได้มากขึ้น อีกทั้งยังใช้ AI ได้มาก ตัวอย่างเช่น ข้อมูลการใช้งาน Character.AI ซึ่งเป็น AI ที่จำลองการสร้างลักษณะบุคลิกต่างๆ เพื่อให้ผู้คนได้เข้าไปใช้งาน พบว่ากลุ่มคนอายุน้อยจะเข้าถึงมากที่สุด และลดหลั่นกันลงไปตามลำดับ
นอกจาก General GPTs ต่างๆ เช่น ChatGPT, Bing, Bard, Claude … จะพบว่ายังมีการใช้ AI ที่เป็นลักษณะทางด้านต่างๆ มากขึ้น
ข้อมูลจากการสำรวจเรื่องการนำ Generative AI ไปใช้ในงานด้านไหนบ้าง ซึ่งถูกเขียนลงใน Blog โดย Marc Zao-Sanders เมื่อวันที่ 19 มีนาคม 2024 3 พบว่า Generative AI ถูกใช้ไปมากที่สุดในด้าน Generating ideas ซึ่งตรงตามวัตถุประสงค์ของการใช้ Generative AI ส่วนด้านอื่นๆ ที่น่าสนใจนำเสนอดังรูปด้านล่าง

จากข้อมูลดังกล่าวข้างต้น AI ถูกใช้ไปในด้านต่างๆ มากมาย นอกจากนี้แนวโน้มการทำงานสมัยใหม่ โดยเฉพาะผู้คนในยุคใหม่ มักจะใช้ AI ร่วมในการทำงานมากขึ้น เพราะฉะนั้นการเรียนรู้ที่จะนำ AI มาใช้ในด้าน แพทยศาสตร์ศึกษา (Medical Education) จึงเป็นสิ่งจำเป็นที่หลีกเลี่ยงไม่ได้
What is Generative AI
Generative AI or generative artificial intelligence refers to the use of AI to create new content, like text, images, music, audio, and videos. - Google4
Generative AI refers to deep-learning models that can generate high-quality text, images, and other content based on the data they were trained on. - IBM 5
จากความหมายข้างต้นหากสรุปรวมจะได้ใจความว่า Generative AI คือ AI ที่สามารถสร้างข้อมูลใหม่ๆ ได้ ไม่ว่าจะเป็น ข้อความ (text), รูปภาพ (images), เสียง (audio) หรือแม้กระทั่งภาพเคลื่อนไหว (videos) ซึ่งเกิดจากการฝึกสอนแบบจำลองด้วยข้อมูลต่างๆ เพื่อให้เกิดเป็น AI ที่สามารถสร้างสรรค์สิ่งเหล่านี้ได้
หากจะลงรายละเอียดให้เข้าใจ Generative AI มากยิ่งขึ้นอาจจะต้องลงไปในรายละเอียดพื้นฐานของเรื่อง AI กันอีกสักนิด
What is AI
Artificial intelligence is the intelligence of machines or software, as opposed to the intelligence of living beings, primarily of humans - Wikipedia 6
Artificial intelligence, or AI, is technology that enables computers and machines to simulate human intelligence and problem-solving capabilities. - IBM 7
จากความหมายของ Wikipedia และ IBM ข้างต้น หากสรุปรวมความหมายให้เข้าใจง่ายๆ คือการที่คอมพิวเตอร์มีความสามารถในการคิดประมวลผลได้เหมือนมนุษย์ เพื่อให้เข้าใจเกี่ยวกับ AI มากยิ่งขึ้น เราจึงควรทำความเข้าใจเล็กๆ น้อยๆ เกี่ยวกับที่มาที่ไป ของ AI รวมถึง พัฒนาการ การสร้างแบบจำลองต่างๆ แบบคร่าวๆ
History of AI
จุดเริ่มต้นของการใช้คำว่า AI จะเป็นเรื่องของการใช้เครื่องจักรในการคำนวณ จนกระทั่งมาในปี 1950 นักคณิตศาสตร์ชาวอังกฤษ Alan Turing 8 ได้มีการเสนอวิธีทดสอบว่าคอมพิวเตอร์สามารถคิดได้เหมือนมนุษย์ได้หรือยัง โดยวิธีการที่เรียกว่า Turing Test

Turing Test
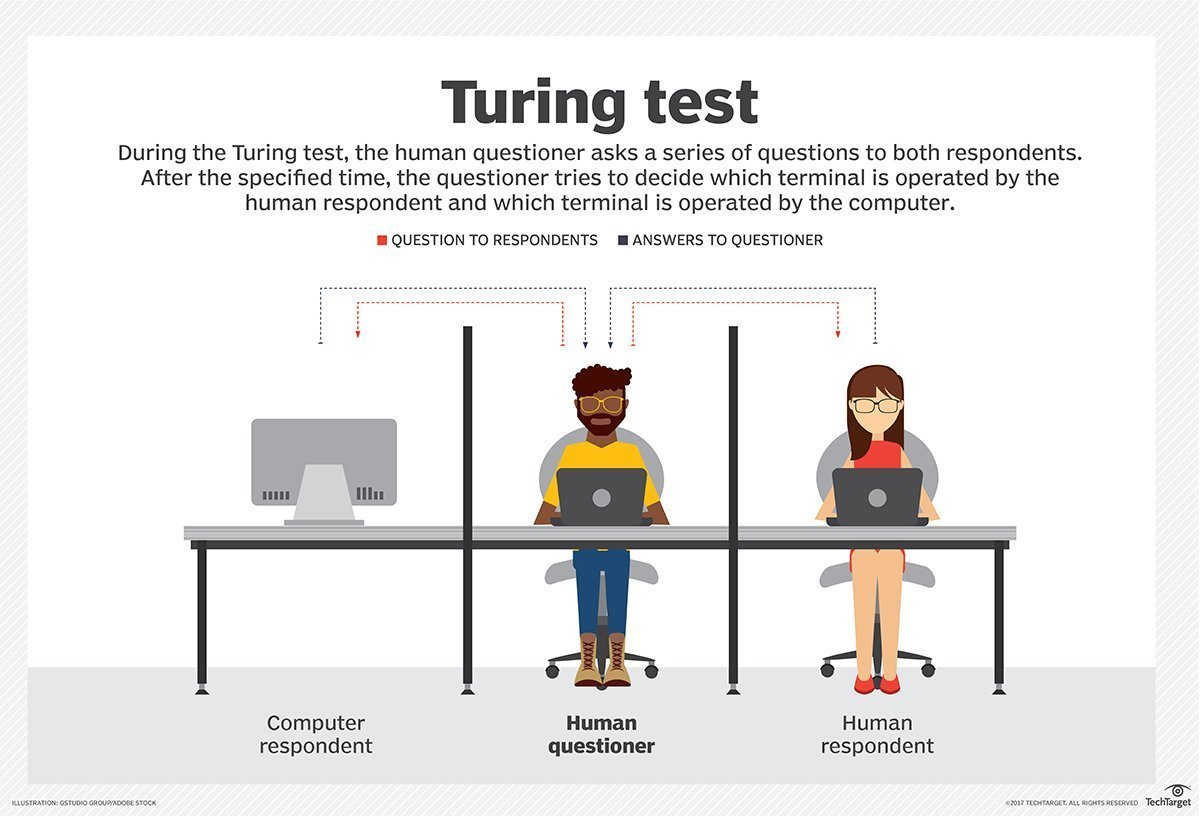
เป็นวิธีการทดสอบที่ให้ผู้ถามคำถาม 1 คนเป็นคนเลือกว่าคำตอบใดเป็นคำตอบที่มนุษย์ตอบ โดยฝั่งผู้ตอบจะมีทั้งมนุษย์และคอมพิวเตอร์เป็นผู้ตอบคำถามเดียวกัน หากผู้ถามคำถาม ไม่สามารถแยกแยะได้ว่าคำตอบใดเป็นของมนุษย์ หรือคอมพิวเตอร์ให้ถือว่าคอมพิวเตอร์มีปัญญาประดิษฐ์ที่มีความสามารถเทียบเคียงมนุษย์ 9
Types of AI
เราสามารถแบ่ง AI ได้หลากหลายวิธี เช่น แบ่งตามความสามารถ, แบ่งตามการทำงาน และแบ่งตามเทคโนโลยี เป็นต้น 10
แบ่งตามความสามารถ
- Narrow AI (Weak AI) ถูกพัฒนามาเพื่อทำงานเพียงไม่กี่อย่าง เช่น การค้นหาข้อมูล, การขับรถ, เล่นเกม เป็นต้น
- General AI (Strong AI) ทำงานและมีความสามารถคล้ายมนุษย์ สามารถแก้ปัญหาที่ไม่เคยเจอมาก่อนได้ โดยใช้ความรู้ที่ตัวเองเคยเรียนมา
- Superintelligent AI เป็น AI แห่งอนาคต ที่จะมีความฉลาดมากกว่ามนุษย์ในทุกๆ ด้าน (ณ ปัจจุบันยังไม่มีข้อมูลว่ามี AI ที่พัฒนาถึงระดับนี้)
แบ่งตามเทคโนโลยี
- Machine Learning ระบบที่สามารถพัฒนาตนเองได้ โดยไม่ต้องมีการโปรแกรมระบบโดยตรง ใช้ข้อมูลสร้างการเรียนรู้
- Deep Learning เป็น subset ของ Machine Learning ซึ่งจะใช้ลักษณะเลียนแบบระบบประสาทของมนุษย์ในการเรียนรู้ (Neural networks) ข้อมูลในการเรียนรู้จะสามารถใช้ข้อมูลปริมาณมหาศาลในการฝึกสอนได้ ส่วนใหญ่จึงมักจะเป็นข้อมูลจำพวก เสียง รูปภาพ หรือประเภทที่ต้องอาศัยข้อมูลปริมาณมากๆ
- Natural Language Processing (NLP) AI ที่เข้าใจภาษามนุษย์ สามารถเป็น Chatbot หรือแปลภาษาได้
- Robotics ตรงไปตรงมาตามชื่อคือ หุ่นยนต์ ทำงานได้หลากหลายประเภทโดยมีลักษณะของ sensory feedback และ informaiton processing
- Computer vision เทคโนโลยีที่สามารถเข้าใจความหมายของสิ่งที่คอมพิวเตอร์เห็น เช่น รูปภาพ สถานที่ หรือแม้กระทั่งแปลผลภาพต่างๆ
- Expert Systems มักจะเป็น rule-based system เป็น AI เฉพาะทางเพื่อตอบปัญหาที่เป็นปัญหาเฉพาะทาง
AI, Machine Learning and Deep learning
เป็นศัพท์ที่ได้ยินบ่อยมากขึ้นในปัจจุบัน ที่จริงแล้วทั้งสามคำนี้มีความเกี่ยวข้องกันโดย AI จะเป็นภาพกว้างๆ ส่วนวิธีการสอน AI ด้วย Machine learning เป็นวิธีหนึ่งในการสร้าง AI เช่นเดียวกับ Deep learning ที่เป็นวิธีย่อยลงไปอีกของ Deep learning ดังภาพ11
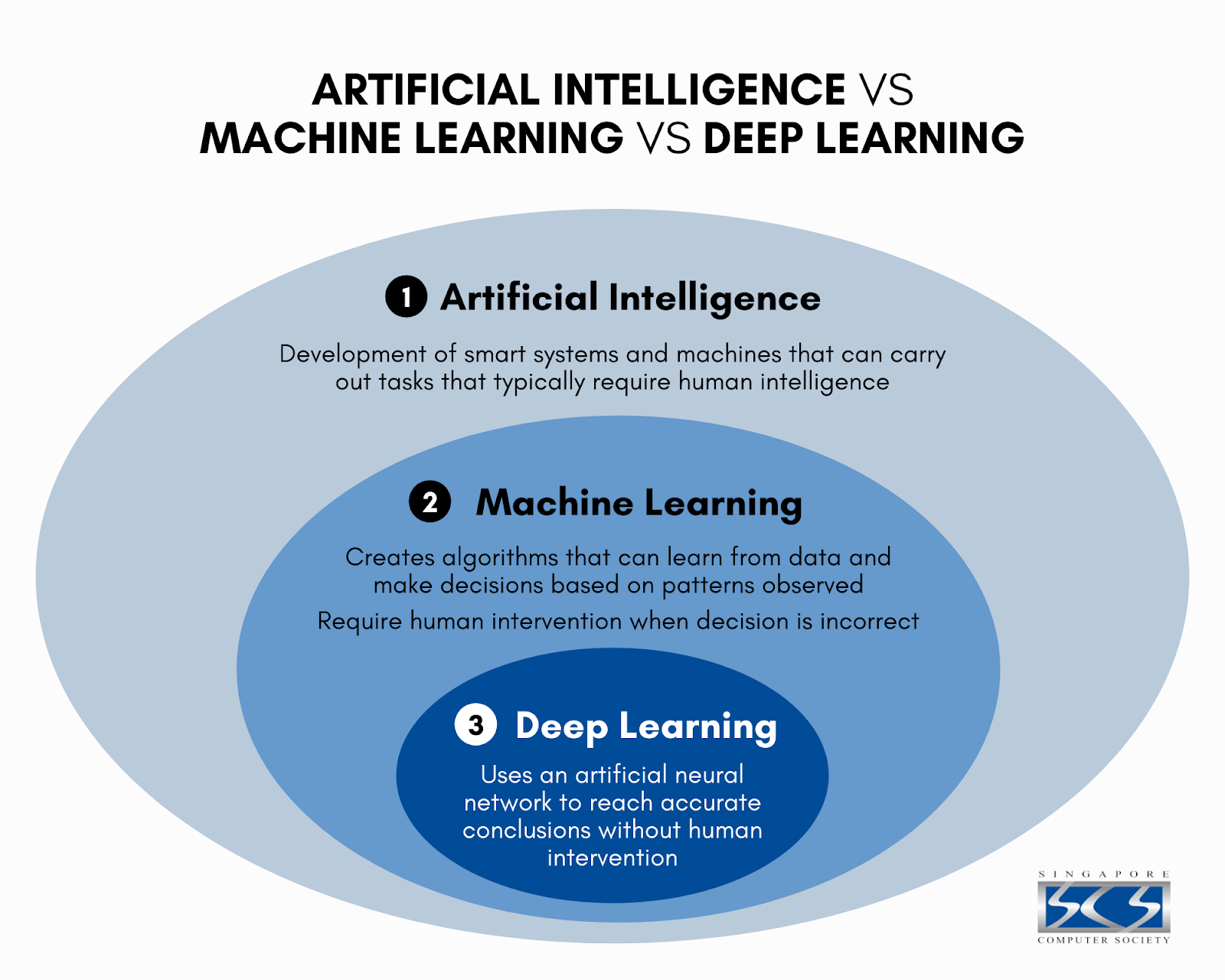
Machine learning
ส่วนหนึ่งของ AI ดังกล่าวข้างต้น โดยการสร้างจะต้องได้รับการสอนผ่านชุดข้อมูลที่มีการเตรียมอย่างเป็นรูปแบบ และนำไปใช้ในการสร้างการตัดสินใจหรือทำนายผลลัพธ์
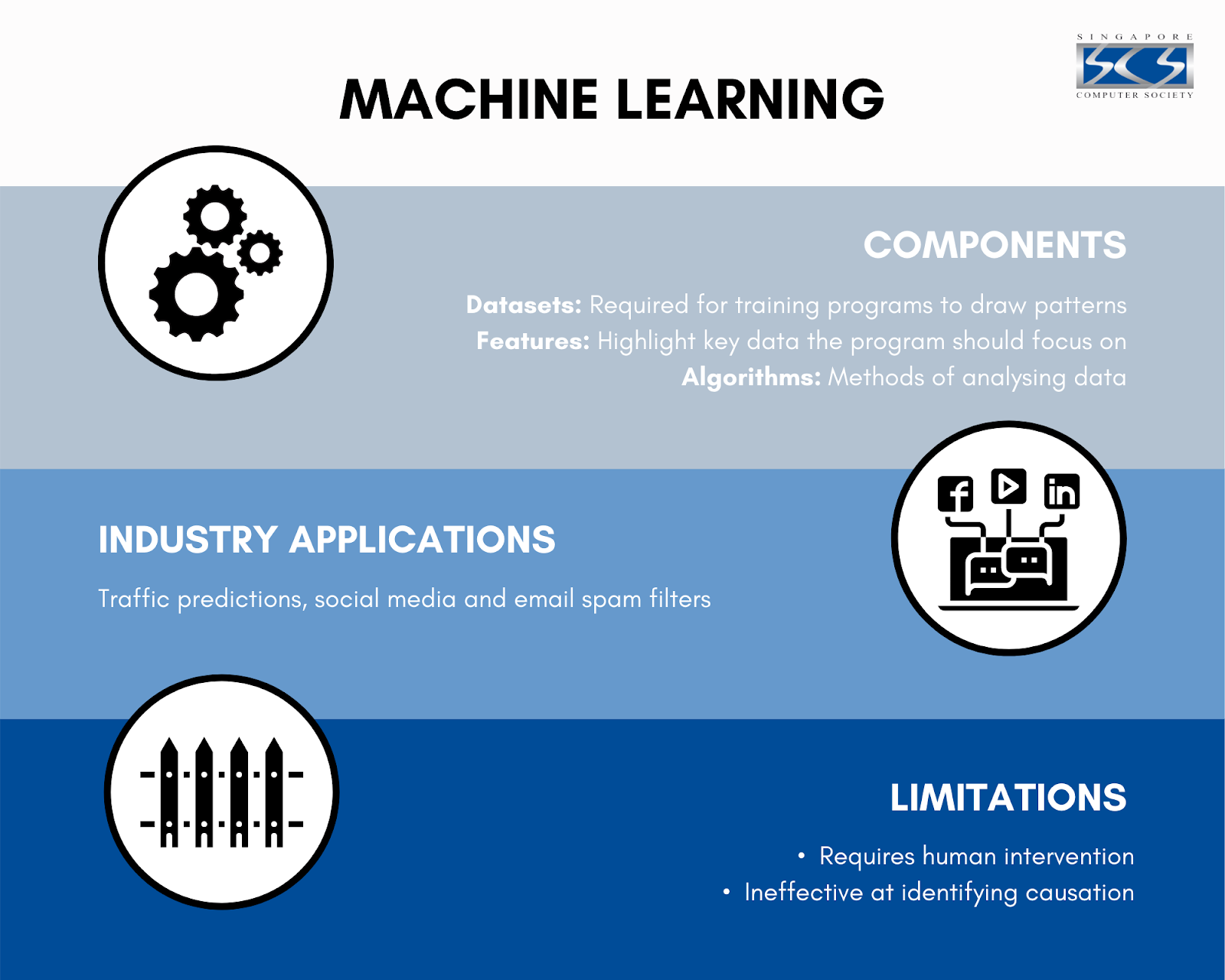
Deep learning
เป็นส่วนหนึ่งของ Machine learning อีกครั้งหนึ่ง ดังนั้น concept ยังคงเหมือนกับ machine learning แต่เพิ่มความซับซ้อนในการสร้างแบบจำลอง โดยการใช้ “Neural network” ซึ่งมักจะใช้ 3 ชั้นขึ้นไป
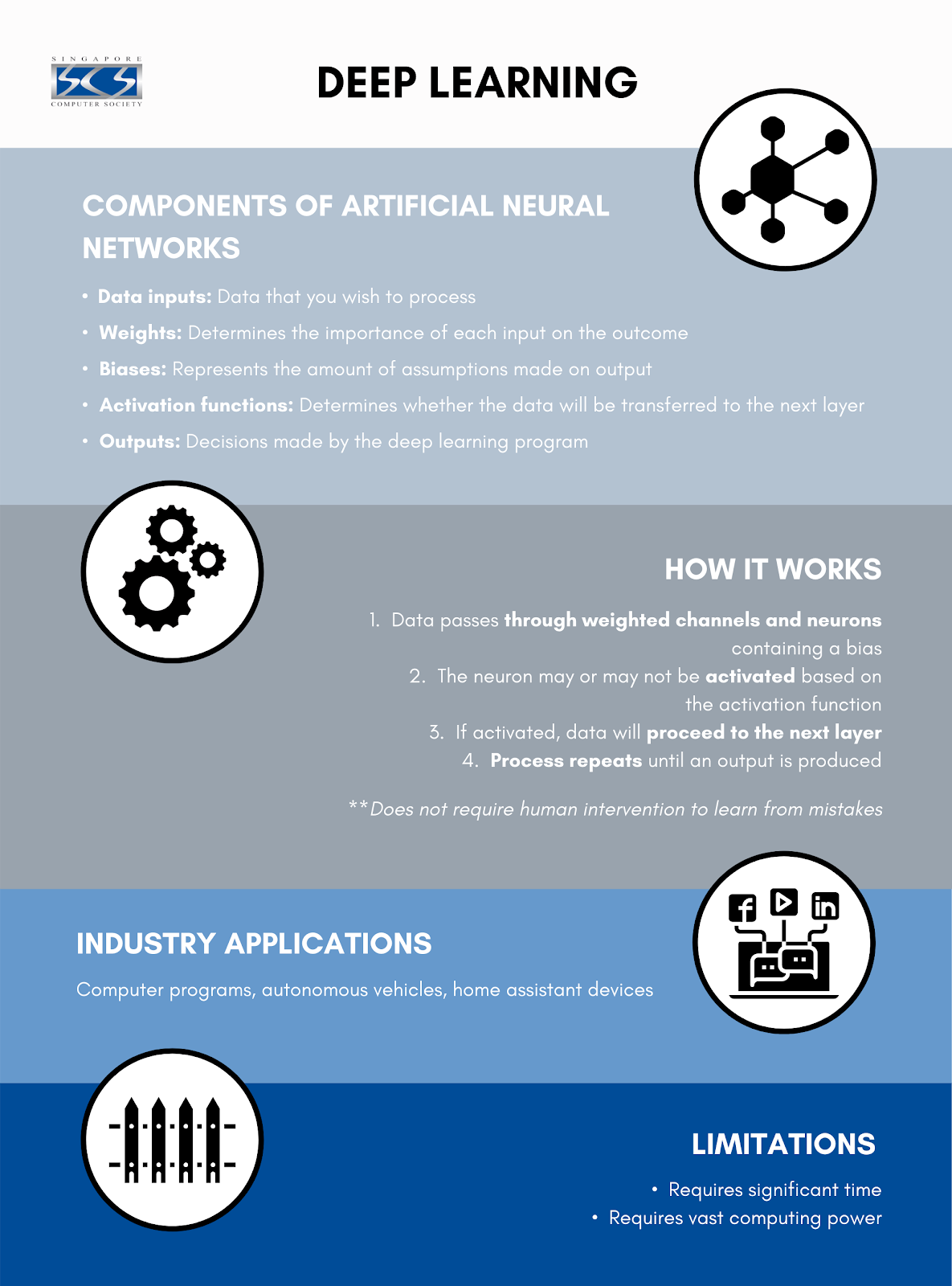
NLP
NLP - Natural language processing คือกระบวนการที่คอมพิวเตอร์ทำความเข้าใจกับภาษาของมนุษย์ เนื่องจากเรื่องของ “ภาษา” นอกจากมีความหลากหลายแล้ว ยังไม่ใช่เป็นลักษณะ Logic เหมือนตัวเลขอย่างที่คอมพิวเตอร์เข้าใจ การที่จะทำให้คอมพิวเตอร์เข้าใจภาษามนุษย์ได้นั้นจำเป็นจะต้องมีการฝึกสอน 12
แล้วคอมพิวเตอร์จะสามารถเข้าใจภาษามนุษย์ได้อย่างไร ลองมาดูนิทานที่จะช่วยให้เข้าใจได้ง่ายขึ้นผ่าน The Octopus Thought Experiment 13
ให้ลองจินตนาการว่ามีคนสองคน ติดเกาะร้างอยู่คนละเกาะ โดยจะแทนชื่อว่า “A” และ “B” ทั้ง A และ B เป็นคนที่พูดภาษาอังกฤษทั้งคู่ ต่างพยายามหาวิธีในการสื่อสารซึ่งกันและกัน และในความโชคร้ายนั้นยังมีความโชคดีที่ทั้งคู่บังเอิญไปพบกับสายโทรเลขเก่าๆ ที่ใช้สื่อสารระหว่างเกาะสองแห่งนี้ ทั้ง A และ B จึงเริ่มสื่อสารกันผ่านสายโทรเลขนี้โดยใช้รหัส “Morse”
Morse หรือรหัสมอส สามารถส่งผ่านสายโทรเลขได้โดยการใช้แรงสั่นสะเทือน แทนรหัส จุด (dot) และ ขีด (dashes) เพื่อนำไปแปลเป็นภาษาอังกฤษอีกครั้งหนึ่ง
วันหนึ่งมีปลาหมึกยักษ์จอมฉลาดตัวหนึ่ง ได้เข้ามาลองสัมผัสสายโทรเลขระหว่าง A กับ B แม้ว่าปลาหมึกตัวนี้จะไม่เข้าใจภาษาอังกฤษเลย แต่ก็ยังรับรู้ถึงแรงสั่นสะเทือนที่พูดคุยกันของ A และ B นานวันเข้าปลาหมึกยักษ์ตัวนี้ก็เริ่มจับลักษณะการสั่นสะเทือนที่เป็นลักษณะการส่งไปมา จนเริ่มที่จะทำนายการตอบสนองของคำถามระหว่าง A และ B ได้ เช่น เมื่อ A ถาม B เกี่ยวกับ ผลไม้บนเกาะของ B ก็มักจะได้รับคำตอบจาก B ที่พูดถึง กล้วย, มะพร้าว หรือน้อยหน่า ปลาหมึกตัวนี้ได้เรียนรู้แนวโน้มของคำต่างๆ ที่จะเกิดขึ้นในบทสนทนาระหว่าง A กับ B
หลังจากที่ปลาหมึกเริ่มรู้สึกเหงา และอยากเล่นสนุนขึ้นมา ปลาหมึกยักษ์จึงตัดสินใจตัดสายโทรเลขออก เพื่อตัดการสื่อสาร และแสร้งทำว่าตนเองเป็น B คุยกับ A โดยที่ A ไม่รู้เรื่องเลย จึงนำไปสู่คำถามว่าจะมีสถานการณ์ใดบ้างที่ A โดนหลกอย่างสมบูรณ์แบบ
จะสังเกตว่าปลาหมึกทำตัวเหมือน Chatbot ที่เรารู้จักกันในปัจจุบัน ลองมาดูตัวอย่าง (อ้างอิงเป็นภาษาอังกฤษเพื่อความเข้าใจ)
“I found ripe guava on my island today which tasted so juicy”, A telegrams.
“Sounds delicious!” the Octopus replies.
หากประเมินการตอบของปลาหมึกในสถานการณ์นี้จะพบว่าสามารถเข้าใจได้ แต่ไม่มีความหมายสำหรับปลาหมึก
ลองตั้งสมมติฐานว่าคำว่า guava จากสถานการณ์ตัวอย่าง เป็นคำศัพท์ที่ไม่เคยผ่านการส่งจาก A ไป B หรือ B ไป A มาก่อน ปลาหมึกยักษ์ของเราก็สามารถหาคำที่สอดคล้องกันจากการตอบของ B ในอดีต เมื่อพบว่ามีคำเหล่านี้อยู่ในประโยคที่ A เคยถาม เช่น ripe, _taste, และ juicy
จากสถานการณ์ข้างต้นจะเริ่มสังเกตพบข้อจำกัดของปลาหมึกยักษ์เมื่อ A ถามคำถามที่ค่อนข้างสร้างสรรค์ ที่คำตอบจะค่อนข้างเป็นลักษณะนามธรรม และความเข้าใจในบริบทของโลกภายนอก
A: “Give me advice on how to build a gigantic catapult.”
A: “There is a monkey attacking me, what do recommend that I do?”
คำถามข้างต้นเป็นคำถามที่ปลาหมึกยักษ์ไม่สามารถตอบได้ ถ้าไม่เคยเห็นคำถามเหล่านี้มาก่อน
ปลาหมึกยักษ์ในเรื่องนี้เป็นตัวแทน Language models (LMs) เช่น GPT-3, BERT, T5 เป็นต้น
Language Model vs. Human Understanding
หัวใจหลักของความแตกต่างในการเข้าใจภาษาระหว่าง LM และมนุษย์ คือ มนุษย์จะมีความเชื่อมโยงภาษากับประสบการณ์ชีวิตจริง แต่ LM จะใช้การประกอบตัวอักษร ขึ้นเป็นข้อความ ตามบริบทที่ได้รับข้อมูลการฝึกสอน ซึ่งแตกต่างจากมนุษย์ที่รับรู้องค์ประกอบด้านอื่นๆ ดังกล่าว
Medical education
Medical education is education related to the practice of being a medical practitioner, including the initial training to become a physician (i.e., medical school and internship) and additional training thereafter (e.g., residency, fellowship, and continuing medical education). - Wikipedia 14
สรุปความโดยสั้นจากความหมายของ Wikipedia ข้างต้น คือ การเรียนการสอนที่เกี่ยวข้องกับทางการแพทย์
คำถามสำคัญคือ เราจะสามารถนำ Generative AI มาทำอะไรได้บ้างเกี่ยวข้องกับ Medical Education
Using generative AI
Generative AI ที่ใช้บ่อยในปัจจุบันมักจะเป็น text-based คือ รับข้อมูลเป็น ข้อความและสร้างข้อความตอบกลับออกมา เช่น ChatGPT 3.5 แต่จะเริ่มมีบางส่วนที่สามารถรับข้อมูลได้ทั้งข้อความและรูปภาพ และสามารถสร้างข้อความหรือรูปภาพตอบกลับออกมาได้ เช่น ChatGPT 4, Gemini เป็นต้น
เพราะฉะนั้นการจะใช้งาน AI ให้สามารถตอบสนองต่อความต้องการได้อย่างถูกต้อง จึงต้องการ Input หรือข้อมูลนำเข้าที่ AI สามารถเข้าใจได้ และตอบสนองตามความต้องการของผู้ใช้งานได้อย่างถูกต้อง สิ่งนี้เรียกว่า “prompt”
Writing good prompt
การเขียนคำสั่ง หรือ prompt ที่ดีสำหรับให้ AI ตอบสนองความต้องการของผู้ใช้งานนั้น มีศาสตร์ที่เกิดขึ้นมาในยุค Generative AI กำลังเป็นที่นิยมคือ ”prompt engineering” แต่ในที่นี้เราสามารถใช้หลักการอย่างง่ายในการเขียน prompt เพื่อให้ตอบสนองตามที่ต้องการได้โดยต้องประกอบด้วยสิ่งเหล่านี้
- Task ต้องการให้ AI ทำอะไร
- Context บริบทแวดล้อมต่างๆ เช่น สถานที่, ผู้เรียน เป็นต้น
- Examples ตัวอย่างการตอบ (เพื่อสร้างแนวทางการตอบ)
- Persona บุคลิกหรือนิสัยส่วนตัว เพื่อสร้างสรรค์ให้ตรงแนวทางของผู้เขียน
- Format รูปแบบการตอบ
- Tone อารมณ์ของคำตอบ
ตัวอย่าง การเขียนจดหมายขอลาป่วยโดยใช้หลักการข้างต้นกับ ChatGPT 3.5
I am a family physician and must be in the outpatient department tomorrow. I'm having diarrhea after I have some sausage.[Context] Please write me a letter[Format] to leave my work tomorrow due to sickness.[Task] I'm normally polite and kind.[Persona] In the empathic mood.[Tone] starting the letter with "To the Director" and ending with my name W.K.[Examples]
คำตอบจาก ChatGPT 3.5 ด้วย prompt ข้างต้น
To the Director,
I hope this message finds you well. I am writing to inform you that unfortunately, I will be unable to fulfill my duties in the outpatient department tomorrow due to sudden illness. It pains me to have to request this time off, but I find myself in a situation where it is necessary for my well-being and the well-being of those I serve.
Regrettably, I have been experiencing symptoms of gastrointestinal distress, likely stemming from a meal I consumed earlier. This has resulted in significant discomfort and has made it impossible for me to carry out my responsibilities effectively.
As a family physician, I understand the importance of being present and available to provide care to our patients. However, in this instance, I must prioritize my health to ensure that I am able to fulfill my duties competently in the future.
I will make every effort to ensure that any urgent matters are appropriately attended to in my absence and that my patients are informed of the situation. Additionally, I will coordinate with my colleagues to minimize any inconvenience caused by my absence.
I sincerely apologize for any inconvenience this may cause and appreciate your understanding and support during this time. Rest assured, I will do my utmost to recover swiftly and return to work as soon as I am able.
Thank you for your consideration.
Warm regards,
W.K.
Hallucination
AI hallucination is a phenomenon wherein a large language model (LLM)—often a generative AI chatbot or computer vision tool—perceives patterns or objects that are nonexistent or imperceptible to human observers, creating outputs that are nonsensical or altogether inaccurate. - IBM 15
ภาวะ ”Hallucination” ในที่นี้ ถ้าเป็นบุคคลากรทางการแพทย์อาจจะสามารถเข้าใจได้โดยความหมาย คือการหลอนข้อมูลขึ้นมาเอง ซึ่งสามารถเกิดขึ้นในการใช้งาน AI โดยเฉพาะ Generative AI สาเหตุมักเกิดจากการที่ Model หรือ Generative AI พยายามสร้างข้อมูลที่ไม่เป็นความจริง หรือไม่เกี่ยวข้องกันขึ้นมาเพื่อตอบสนองต่อ prompt ของผู้ใช้งาน
เพราะฉะนั้นข้อมูลต่างๆ ที่ถูกสร้างจาก Generative AI จึงไม่สามารถเชื่อถือได้ 100% ต้องมีการตรวจสอบซ้ำเป็นสิ่งสำคัญ ยิ่งหากเราทราบข้อจำกัดของ Generative AI ตั้งแต่การออกแบบ หรือความสามารถอื่นๆ ของ AI ที่กำลังใช้งานจะสามารถระมัดระวังได้มากยิ่งขึ้น เช่น ข้อจำกัดสำคัญของ ChatGPT 3.5 คือ ข้อมูลที่ถูกฝึกสอน AI เป็นข้อมูลที่ update ล่าสุดแค่ มกราคม ปี 2022 และอีกข้อจำกัดสำคัญคือ model ไม่สามารถเชื่อมต่อ internet ได้ เพราะฉะนั้นจากข้อจำกัดของ Model ดังกล่าวข้างต้นหากมีการใช้งานที่เป็นสิ่งใหม่ๆ เกิดขึ้นหลัง มกราคม ปี 2022 มีแนวโน้มเป็นอย่างยิ่งที่ Model ดังกล่าวจะไม่รู้จักมาก่อน การสร้างข้อมูลออกมาอาจเป็นเท็จได้ (Hallucination)
Plan your teaching
การเตรียมการสอนเป็นขั้นตอนสำคัญ อย่างน้อยจะต้องตอบคำถามสำคัญให้ได้ คือ Who, What, How และ How will i know if students understand? อ่านเพิ่มเติม
สามารถนำ Generative AI มาใช้ได้หลากหลายขั้นตอน โดยใช้ความสามารถในการสร้างสรรค์มาช่วยได้ตั้งแต่ในขั้นตอนการวางแผนการสอน สามารถออกแบบ ตามหลัก “OLE” (Objective, Learning, Evaluation) ได้อย่างครบวงจร โดยการใช้ prompt ที่ดี
_ตัวอย่างการสร้าง Lesson plan เรื่อง Health system Science สำหรับ นศพ.ปี 4 โดยใช้ ChatGPT 3.5
Title: Understanding Health System Science: A Collaborative Exploration
**Objective:**
By the end of this session, students will be able to:
1. Define Health System Science (HSS) and its significance in clinical practice.
2. Identify key components of the health system and their interconnections.
3. Analyze challenges within health systems and propose potential solutions.
4. Apply HSS principles to clinical scenarios.
**Duration: 60 minutes**
**Materials Needed:**
1. Whiteboard or flip chart
2. Markers
3. Handouts with relevant readings on HSS (optional)
**Lesson Plan:**
**Introduction (5 minutes)**
- Welcome students and introduce the topic of Health System Science (HSS).
- Discuss the importance of understanding health systems for future clinicians.
- Explain that the session will be interactive and based on small group discussions.
**Activity 1: Defining Health System Science (10 minutes)**
- Divide students into small groups of 3-4.
- Provide each group with a whiteboard or flip chart.
- Ask each group to brainstorm and define HSS based on their prior knowledge and understanding.
- After 5 minutes, have each group present their definition, and compile key points on the main board.
**Activity 2: Components of the Health System (15 minutes)**
- Provide a brief overview of the components of a health system: delivery, workforce, information, financing, and governance.
- Divide students into new small groups.
- Assign each group one component of the health system.
- Ask groups to discuss the roles, challenges, and interactions of their assigned component within the health system.
- After 10 minutes, reconvene and have each group share their insights.
**Activity 3: Analyzing Health System Challenges (15 minutes)**
- Introduce common challenges within health systems such as access, quality, equity, and cost.
- Assign each group one challenge.
- Instruct groups to brainstorm potential causes of the assigned challenge and propose solutions.
- Encourage creative thinking and consideration of real-world examples.
- After 10 minutes, have each group present their analysis and solutions.
**Activity 4: Applying HSS to Clinical Scenarios (15 minutes)**
- Provide clinical scenarios related to health system issues (e.g., patient unable to afford medication, long wait times for specialist appointments).
- Divide students into pairs or small groups.
- Instruct them to analyze the scenario using HSS principles discussed earlier.
- Encourage discussion on how understanding health systems can inform clinical practice and decision-making.
- After 10 minutes, reconvene and allow each group to share their analysis and proposed actions.
**Conclusion (5 minutes)**
- Summarize key points discussed during the session.
- Emphasize the importance of integrating HSS knowledge into clinical practice.
- Invite students to reflect on how they can apply HSS principles in their future careers.
- Provide resources for further reading or exploration on the topic.
**Wrap-Up:**
- Thank the students for their participation.
- Encourage them to continue exploring and applying HSS concepts in their clinical training and future practice.
**Note:**
- It's essential to facilitate discussions and provide guidance as needed throughout the activities to ensure that students stay on track and achieve the learning objectives.
จากตัวอย่างจะเห็นว่า มี Lesson plan ที่เกือบครบสมบูรณ์ สามารถนำไปประยุกต์ใช้ในการเตรียมการสอน โดยผู้สอนอาจจะได้วิธีการสอน หรือไอเดียใหม่ๆ ในการนำไปประกอบการสอน หรือแม้กระทั่งสามารถนำไปลงรายละเอียดในหัวข้อต่างๆ เพิ่มมากขึ้น เช่น สามารถให้ list หัวข้อที่จำเป็นสำหรับการทำ slide wrap-up ดังตัวอย่าง
**Slide: Conclusion**
- Summary of Key Points:
- Defined Health System Science (HSS) and its significance.
- Explored components of the health system: delivery, workforce, information, financing, and governance.
- Analyzed common challenges within health systems: access, quality, equity, and cost.
- Discussed potential solutions and applications of HSS principles in clinical scenarios.
- Importance of Integrating HSS:
- Enhances understanding of healthcare dynamics.
- Improves clinical decision-making.
- Facilitates advocacy for system-level improvements.
- Reflection:
- Encourage students to reflect on how they can apply HSS knowledge in their future clinical practice.
- Invite them to consider how they can contribute to addressing health system challenges.
- Resources for Further Exploration:
- Provide links to relevant readings, articles, or organizations focusing on Health System Science.
- Encourage continued learning and engagement with the topic beyond the classroom.
- Thank You:
- Express gratitude for the students' participation and engagement throughout the session.
- Emphasize the importance of their role in shaping future healthcare systems as informed clinicians.
Assessments
อีกหนึ่งส่วนที่สำคัญสำหรับการเรียนการสอนคือการประเมินผลทั้ง Formative และ Summative ปัญหาหลักๆ ของการประเมินผลสำหรับอาจารย์แพทย์คือ ”การออกข้อสอบ” ซึ่งสามารถใช้ Generative AI ช่วยได้ค่อนข้างมาก ยกตัวอย่างการออกข้อสอบ MCQ
ตัวอย่าง การใช้ ChatGPT 3.5. ออกข้อสอบ MCQ 5 ตัวเลือก เกี่ยวข้องกับศัลยกรรม
Question: Which of the following is a common complication associated with laparoscopic cholecystectomy?
A) Wound infection
B) Bile duct injury
C) Pneumothorax
D) Hypertrophic scar formation
E) Peripheral nerve injury
Correct Answer: B) Bile duct injury
Explanation: Laparoscopic cholecystectomy is a minimally invasive surgical procedure commonly performed for the removal of the gallbladder. Despite its advantages, it carries the risk of bile duct injury, which is a significant complication. Bile duct injuries can lead to bile leakage, bile peritonitis, and subsequent complications such as biliary strictures and liver damage. While wound infection (Option A) is a potential complication of any surgical procedure, it is not specific to laparoscopic cholecystectomy. Pneumothorax (Option C) is a complication associated with thoracic surgeries or procedures involving the chest cavity, not commonly encountered in cholecystectomy. Hypertrophic scar formation (Option D) is more commonly seen in superficial wounds and is not a prominent complication of laparoscopic cholecystectomy. Peripheral nerve injury (Option E) is not a typical complication associated with this procedure. Therefore, the correct answer is B) Bile duct injury.
จากตัวอย่างข้างต้นจะพบว่าสามารถออกข้อสอบ MCQ ได้ แต่อาจจะยังไม่ใช้ลักษณะข้อสอบ MCQ ที่ดี เราสามารถใช้ตัวอย่างที่ Generative AI สร้างออกมาข้างต้น ปรับปรุงด้วย prompt เพิ่มเติม ได้ตามตัวอย่างต่อไป
Question: During a laparoscopic cholecystectomy procedure, the surgeon inadvertently transects a structure near the gallbladder. As a result, bile leakage occurs postoperatively. Which of the following structures is most likely to have been injured?
A) Common hepatic artery
B) Cystic duct
C) Portal vein
D) Common bile duct
E) Superior mesenteric artery
Correct Answer: D) Common bile duct
Explanation: In laparoscopic cholecystectomy, the common bile duct is at risk of injury due to its close proximity to the gallbladder. Transection or injury to the common bile duct during the procedure can lead to bile leakage postoperatively. The common hepatic artery (Option A) supplies blood to the liver but is not typically encountered during cholecystectomy. The cystic duct (Option B) is commonly ligated and divided during the procedure but does not lead to bile leakage if injured. The portal vein (Option C) and superior mesenteric artery (Option E) are not typically encountered during cholecystectomy and are not associated with bile leakage if injured. Therefore, the most likely structure to have been injured in this scenario is the common bile duct (Option D).
จะเห็นว่าเมื่อเราใช้ความสามารถในการจดจำของ Generative AI (การจดจำหมายถึง Generative AI สามารถจำคำตอบและคำถามที่ต่อเนื่องกันมาได้ เสมือนคนคุยกัน) เพิ่มรายละเอียดให้มีบริบทผู้ป่วยและเป็นข้อสอบแนวประยุกต์มากขึ้น ข้อสอบที่ได้ตามตัวอย่างจะเริ่มมีคุณภาพมากขึ้น
ข้อควรระวังคือ อาจารย์จำเป็นจะต้องตรวจสอบความถูกต้องของข้อมูลเสมอ
Scoring systems
สามารถสร้างแนวทางการให้คะแนนได้
ตัวอย่างการสร้างแนวทางการให้คะแนนสำหรับการสอบ OSCE
Assessment Criteria | Points |
---|---|
Introduction and Communication Skills | |
- Introduction and greeting | 5 |
- Effective communication | 10 |
- Informed consent | 5 |
Explanation of the Procedure to the Patient | |
- Clarity of explanation | 10 |
- Addressing patient concerns/questions | 5 |
Proper Technique for NG Tube Insertion | |
- Correct technique | 20 |
- Equipment handling | 10 |
Confirmation of NG Tube Placement | |
- Confirmation methods | 15 |
- Recognition and addressing of incorrect placement | 10 |
Securement of the NG Tube | |
- Proper securing | 10 |
Post-procedural Care Instructions | |
- Clarity of instructions | 10 |
Overall Professionalism and Demeanor | |
- Professionalism and respect | 10 |
- Confidence and competence | 10 |
Total Possible Points: 100
Grading:
- Excellent: 90-100 points
- Good: 80-89 points
- Satisfactory: 70-79 points
- Needs Improvement: 60-69 points
- Inadequate: Below 60 points
This adjusted scoring guide provides a more detailed breakdown of points for each criterion, allowing for a more nuanced assessment of candidate performance.
อาจารย์แพทย์สามารถนำไปประยุกต์ตามแนวทางการสอบ และการให้คะแนนของตนเองได้
Others
นอกจากความสามารถที่ได้สร้างเป็นตัวอย่างข้างต้น ยังสามารถใช้งานช่วยเหลือเพิ่มเติมได้ดี โดยเฉพาะส่วนที่เป็นเรื่องของความคิดสร้างสรรค์ ยกตัวอย่างเช่น การใช้ Generative AI ที่มีความสามารถในการสร้างรูปภาพ อาจารย์สามารถนำมาใช้สร้างรูปภาพประกอบการสอน หรือประกอบ slide นำเสนอได้ หรืออาจจะใช้ช่วยในการแปลภาษา ทั้งการแปลจากภาษาไทย ไปเป็นภาษาอื่นๆ หรือแปลกลับมาเป็นภาษาไทยก็เป็นสิ่งที่ Generative AI สามารถทำได้ดี